Enhancing AI Performance: A Comprehensive Guide to Fine-Tuning and Retrieval-Augmented Generation
- Andrew
- Dec 12, 2024
- 3 min read
Last Edited: December 12, 2024
Editor: Andrew
Introduction
Artificial intelligence is changing how businesses work, and companies want to make their AI tools smarter and more useful. Two main ways to improve AI are called "fine-tuning" and "retrieval-augmented generation" (RAG). Think of these like different ways of teaching a smart robot to do specific jobs better [1].
This guide will break down these methods in simple terms, helping you understand which approach might work best for your needs.
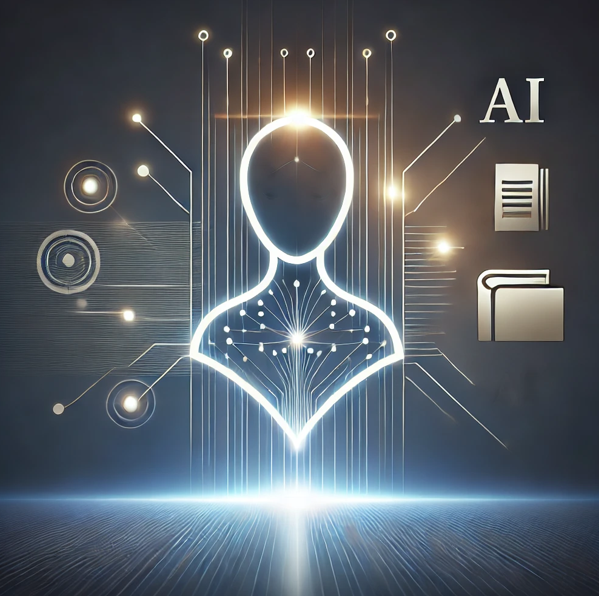
Understanding the Techniques
Fine-Tuning: Specialized AI Training
Fine-tuning is like taking a smart student (an AI model) and giving them extra lessons in a specific subject. Instead of starting from scratch, you build on what the AI already knows and help it become an expert in a particular area[2].
How Fine-Tuning Works:
Gather Special Data: Collect information about a specific topic.
Retrain the AI: Help the AI learn the unique details of this topic.
Create a Specialized Tool: Deploy the newly trained AI for specific tasks.
Real-World Example: A law firm takes a basic AI and teaches it legal language by showing it hundreds of legal documents. Now the AI can draft contracts almost like an experienced lawyer.
Retrieval-Augmented Generation (RAG): Smart Information Gathering
RAG is like giving the AI a powerful research assistant. Instead of memorizing everything, the AI can quickly look up the most recent and relevant information right when it needs to answer a question[3].
How RAG Works:
Build a Knowledge Library: Create a searchable collection of documents.
Find Relevant Info: Quickly search through the library when needed.
Create Smart Answers: Combine the found information with the AI's existing knowledge.
Real-World Example: A customer support chatbot can instantly pull up the latest troubleshooting guides to help solve a customer's specific problem.
Cost and Performance Analysis
Performance Dimensions
Dimension | Fine-Tuning | RAG |
Data Type | Fixed, specific datasets | Flexible, changing information |
Setup Time | Weeks to months | Days to weeks |
Initial Cost Guideline* | $20,000 - $70,000+ | $8,000 - $30,000 |
Updating Difficulty | Hard (needs full retraining) | Easy (quick knowledge updates) |
Ability to Grow | Limited | High |
*Actual costs are project, model, and development specific.
Cost Breakdown Example Guidelines
Initial Setup Costs
Cost Component | Fine-Tuning | RAG |
Data Preparation | $5,000 - $20,000 | $2,000 - $10,000 |
Model Training | $10,000 - $30,000+ | Not needed |
Knowledge Base Setup | Not needed | $500 - $5,000 |
Expertise | $5,000 - $15,000 | $5,000 - $15,000 |
Total | $20,000 - $70,000+ | $8,000 - $30,000+ |
*Actual costs are project, model, and development specific.
Ongoing Costs Example Guidelines
Cost Component | Fine-Tuning | RAG |
Data Updates | $10,000+ per retraining | $500 - $2,000 per update |
Hosting and Scaling | $500 - $5,000/month | $100 - $500/month |
Query Costs | Moderate, token-based | Lower, token-based + retrieval fees |
*Actual costs are project, model, and development specific.
Decision Framework
To determine the optimal approach for your project, consider these key questions:
Data Dynamics:
Is your data predominantly static or rapidly changing?
Fine-tuning excels with stable, well-defined domains.
RAG performs better with dynamic, evolving information.
Computational Resources:
Assess your budget and computational capacity.
Fine-tuning requires significant upfront investment.
RAG offers more flexible, cost-effective scaling.
Performance Requirements:
Prioritize precision vs. adaptability.
Fine-tuning delivers high accuracy in narrow domains.
RAG provides flexibility across broader contexts.
Hybrid Approaches: Combining Strengths
Modern AI strategies increasingly explore hybrid models that leverage both fine-tuning and RAG. These approaches allow organizations to:
Maintain core model capabilities through fine-tuning.
Enhance responsiveness with dynamic knowledge retrieval.
Customize AI behavior across different application contexts.
Precedence and Knowledge Integration
When combining techniques, several factors influence how retrieved and fine-tuned knowledge interact:
Prompt design
Retrieval system configuration
Completeness of external knowledge
Specific implementation details
Ethical and Practical Considerations
Potential Challenges
Ensuring data quality and representation
Mitigating potential biases in training data
Maintaining consistent model performance
Balancing computational efficiency with model complexity
Future Outlook
Emerging trends suggest continued innovation in AI model enhancement:
More sophisticated hybrid approaches
Advanced semantic search techniques
Improved methods for dynamic knowledge integration
Enhanced computational efficiency
Conclusion
Fine-tuning and RAG represent powerful, complementary strategies for AI enhancement. While no universal solution exists, understanding their strengths allows organizations to make informed decisions.
For most businesses, a pragmatic approach involves:
Starting with RAG for initial implementation.
Exploring fine-tuning for specific, high-precision use cases.
Continuously evaluating and adapting your AI strategy.
References
[1] Brown, T. B., et al. (2020). "Language Models are Few-Shot Learners." arXiv preprint arXiv:2005.14165.
[2] Devlin, J., et al. (2018). "BERT: Pre-training of Deep Bidirectional Transformers for Language Understanding." Proceedings of NAACL-HLT, 4171-4186.
[3] Lewis, P., et al. (2020). "Retrieval-Augmented Generation for Knowledge-Intensive NLP Tasks." Advances in Neural Information Processing Systems, 33, 9459-9474.
Note: This guide provides a general overview. Specific implementation details should be tailored to your organization's unique requirements and technological infrastructure.